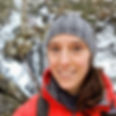
Emma Strubell is an Assistan Professor at the Language Technologies Institute in the School of Computer Science at Carnegie Mellon University (CMU), and a Visiting Scientist at the Allen Institute for Artificial Intelligence. Previously she held research scientist roles at Google and FAIR after earning her doctoral degree in 2019 from the University of Massachusetts Amherst. Her research lies at the intersection of natural language processing (NLP) and machine learning (ML), with a focus on green (computationally efficient) AI and providing pragmatic solutions to practitioners who wish to gain insights from natural language text. Her work has been recognized with a Madrona AI Impact Award, best paper awards at ACL 2015 and EMNLP 2018, and cited in news outlets including the New York Times and Wall Street Journal.
Strubell’s research is broadly motivated by the goal of democratizing AI (specifically, NLP). For Strubell this means that everyone should be able to shape, and shape uses of, NLP technology, with or without access to substantial compute resources; and the NLP community should provide text analysis solutions that are useful across a broad array of applications for solving real-world problems, not just tasks with the highest potential to increase capital. She leads a research group at CMU that aims to push the NLP community towards these goals, which manifests in a variety of more specific research directions, including computation- and data-efficient machine learning for NLP, transfer learning and generalization, NLP for expert domains such as scientific articles and legal text, structured prediction, and ethical issues in ML and NLP.
Strubell is most well known for her 2019 article “Energy and policy considerations for deep learning in NLP,” which estimated the carbon footprint of energy for training some modern AI models, finding that these emissions can be non-trivial, potentially amounting to more than 2x the carbon footprint of a car over its lifetime, including fuel. Following that work, she co-authored (with nDEE researcher Lynn Kaack and others) the article “Aligning artificial intelligence with climate change mitigation,” which outlines a framework describing the effects of machine learning on GHG emissions, published in Nature Climate Change. She has also co-authored articles exploring the relationship between fairness and environmental sustainability in NLP, and proposing methods by which users of cloud compute services can reduce the carbon footprint of their energy use by more flexible scheduling of compute workloads. She has also been involved in professional service aimed at promoting research into computational efficiency and “green AI,” co-organizing a working group in the ACL, the premier technical society and publisher for the field of NLP, that succeeded in implementing policy changes towards that end.